Case study of Firestone UK (Bridgestone) with Springbok Agency
The company & project
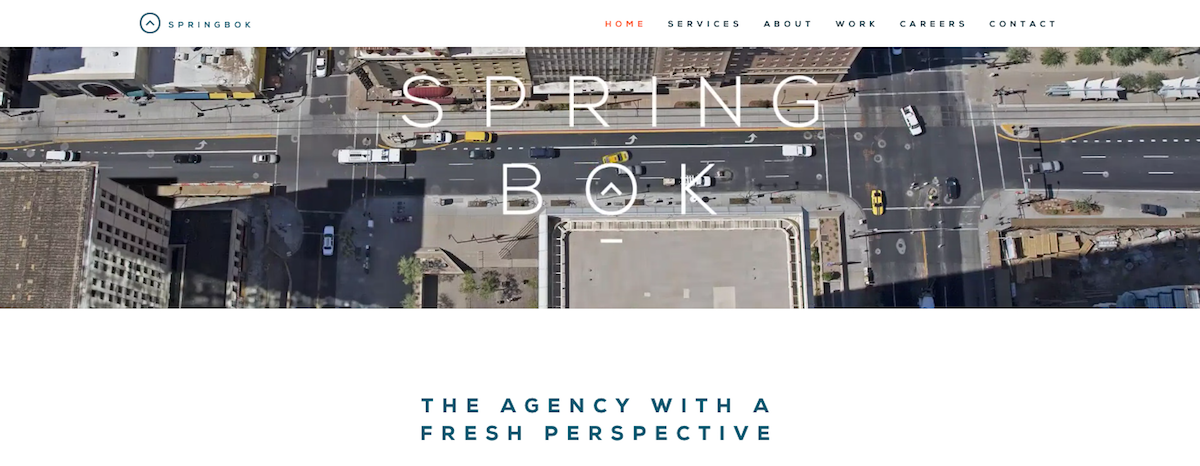
Springbok Agency website
Springbok is a Belgian based digital agency that provides digital services for companies in need of a digital strategy, marketing consulting, or specific technical support. Springbok aims to be at the forefront bringing in disruptive ideas and solving technical challenges.
That’s why, when Bridgestone asked Springbok to improve the website of their brand “Firestone”, they didn’t go the easy way. They went a step further by offering to build a chatbot. And that made especially sense for that project as you’re going to discover it now.
The challenge of the chatbot
Firestone’s website has two clear goals:
- entice the visitor to choose new tyres for his car (section “Find a tyre”)
- or find a vendor to buy them (section “Find a dealer”)
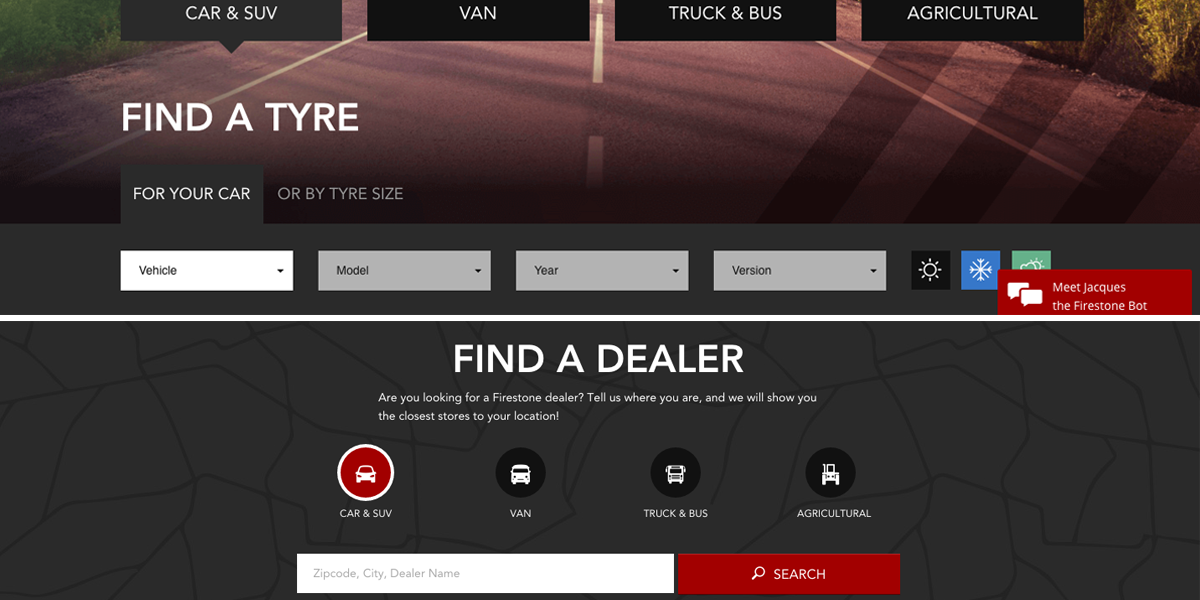
Firestone UK website has 2 very clear goals
This is more complicated than it seems.
Finding the right tyres for a car is quite difficult because a lot of parameters come into account. The current process requires visitors to go through a long series of drop-down menus to introduce all the required information about their vehicle or tyre’s reference.
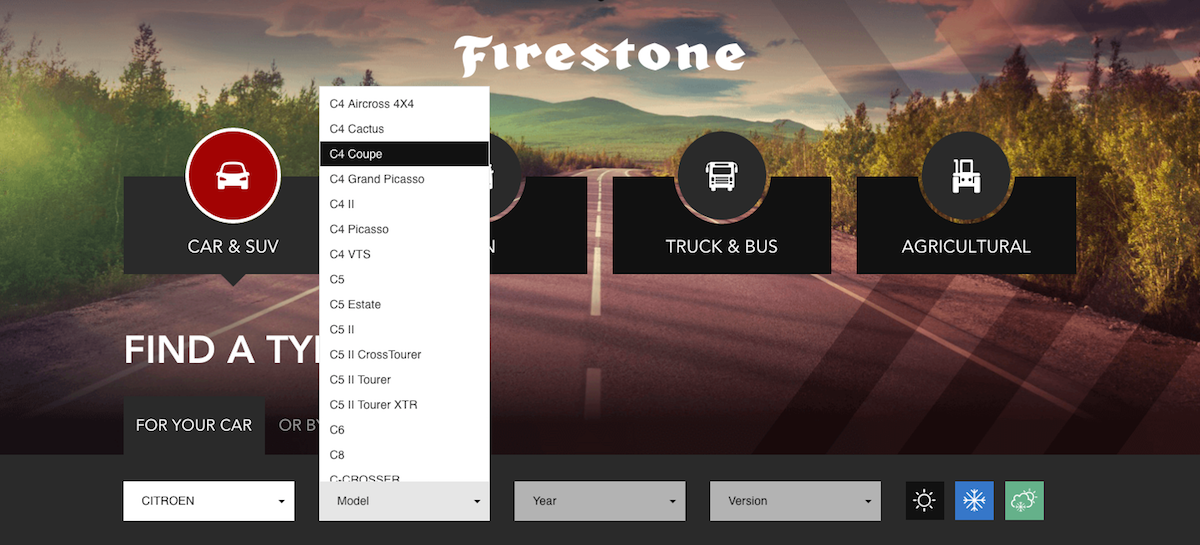
Drop-down menus on Firestone UK website
In that case, a chatbot is a very appropriate solution to ease the pain of a long, time-wasting process. Potentially it can guide visitors through the whole experience in a helpful and easy way. Giving information – even specific ones – during a conversation should make it a much more enjoyable experience than filling in a form. Should. If it works well.
Alexis Safarikas, the digital strategist at Springbok, is the person who brought the idea of a chatbot replacing the form on the website. Once the project was accepted, he quickly realised that he had to ensure that the chatbot he was building for Bridgestone was indeed working well. To do so, Alexis had in mind a few important KPIs he wanted to measure.
What Springbok wanted
A chatbot is a conversational interface. The users of a chatbot have a conversation with a robot to achieve something: this is called the intent. It is what your users intend to do with your chatbot, what they want to achieve. Chatbots can have closed or open intents.
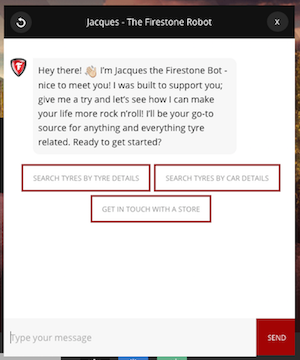
Firestone chatbot – choosing one of the intents
In Bridgestone’s case, of course, the chatbot has closed intents. Bridgestone knows exactly what they want their users to achieve with the chatbot.
3 simple intents were identified:
- Find tyres through car information
- Find tyres through tyre characteristic
- Find and contact a dealer
The most important KPI Alexis wanted to get was the success rate for each of the intents. That, according to him, would give a good indication on how well the chatbot itself was working. He was also eager to know the duration of each chat, the click-through rate of the links to vendors… But how to get these numbers? There was no easy answer because, unfortunately, there aren’t too many analytics around to help with that.
Until Springbok came in contact with UXprobe.
UXprobe’s contribution
UXprobe has developed an online software to analyse User Experience by measuring success and satisfaction in apps, websites, but also in chatbots.
Therefore UXprobe brought a solution perfectly suited to Springbok’s needs and requirements.
- Definition of goals and measurements: UXprobe starts as a service with an evaluation of the intents to make sure you get the appropriate metrics
- Easy to connect to REST service: UXprobe works with all the existing tools and NLP service that are already implemented.
- Automatic collection of data both quantitative and qualitative
- Analysis in UXprobe’s dashboard: Online dashboard displaying a UX metrics overview with relevant graphs and charts.
Numbers are in
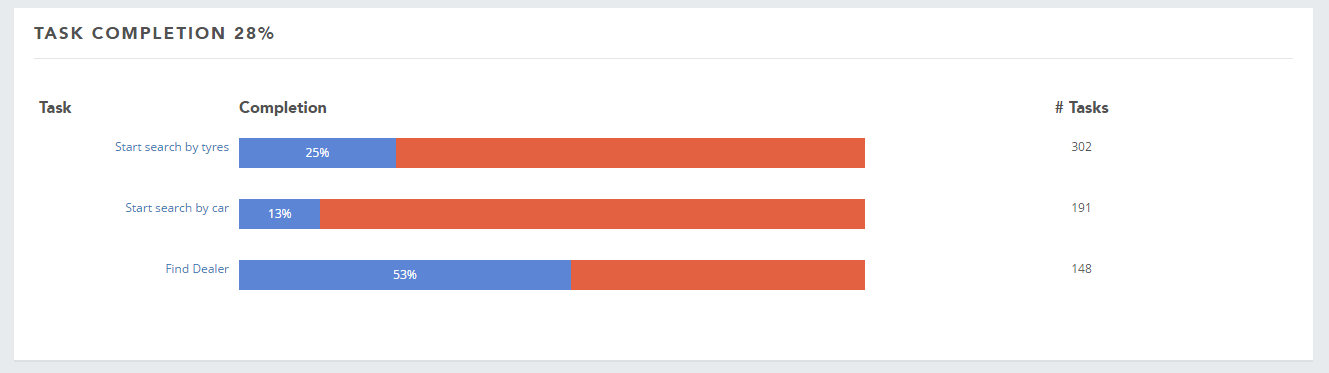
Task completion graph in UXprobe dashboard:
Start search by tyres: 25% completion – Start search by car: 13% completion – Find dealer: 53% completion
The base unit to measure the user experience (UX) is a task; it is the equivalent of the intent. It is then trivial to use UX metrics based on tasks to measure the quality of chatbots by using the intent as the base unit.
It was therefore very easy to get the numbers that Alexis was so eager to collect: simple but critical KPIs to measure the quality of the chatbot:
- Success rate on 3 different intents: users reaching the end of the chat with the right type of tyres, that’s a success!
- Chat duration: how long does it take to successfully complete a chat
- Click-Through-Rate: how many users click on the button “Contact the dealer”
- Satisfaction rate: quick feedback panel at the end to rate the experience on a scale from 1 to 5
- Specific bugs identification: tagged as error when a session is too long when the chat cannot understand the users’ answer/language
With these KPIs in hand, the possibility to compare them with the existing website and with new iterations of the chatbot as they come in, Alexis has control over the chatbot he’s building for Bridgestone.
Results and learnings
The success rates show that the chatbot is more successful when the search starts by tyres than by type of car (25% success against 13%). The number of visits shows also that more users choose to search by tyres (262 users against 166 for search by car). The success rate for finding a dealer is much higher: 53%.
The drop-off rates range from 40.45% (search by tyre), 31.92% (search by car) to 21.95% (dealer locator).
At the end of the chat, 24 users clicked to request contact information from dealers.
The qualitative survey got 15 answers (3x “3stars” + 7x “4 stars” + 5x “5 stars”), and Firestone received 2 emails feedback: “cool” + “I like this bot!”.
The comparison with the existing website showed that visitors were preferably using the chatbot for searching tyres, and the website for searching for a dealer. The conversion rates for finding a dealer were almost the same (19.5% for the chatbot, 21.51% for the website).
The drop-off rates and some other errors that were identified thanks to UXprobe suggested that the quality of the chatbot was not optimal. After investigation, Springbok saw that the problems were related to the reliability of the API service they were using. Detected soon enough, they could find a solution in a short period of time.
Even if there is still room for improvement at this point, Alexis is now confident to tell his client that he’s driving the new chatbot towards success.
Do you also want to improve the quality of your chatbot? Contact Us.
ABOUT THE AUTHOR
Isabelle Dro
Digital Marketer